UNESCO calls for regulations on use of GenAI in schools
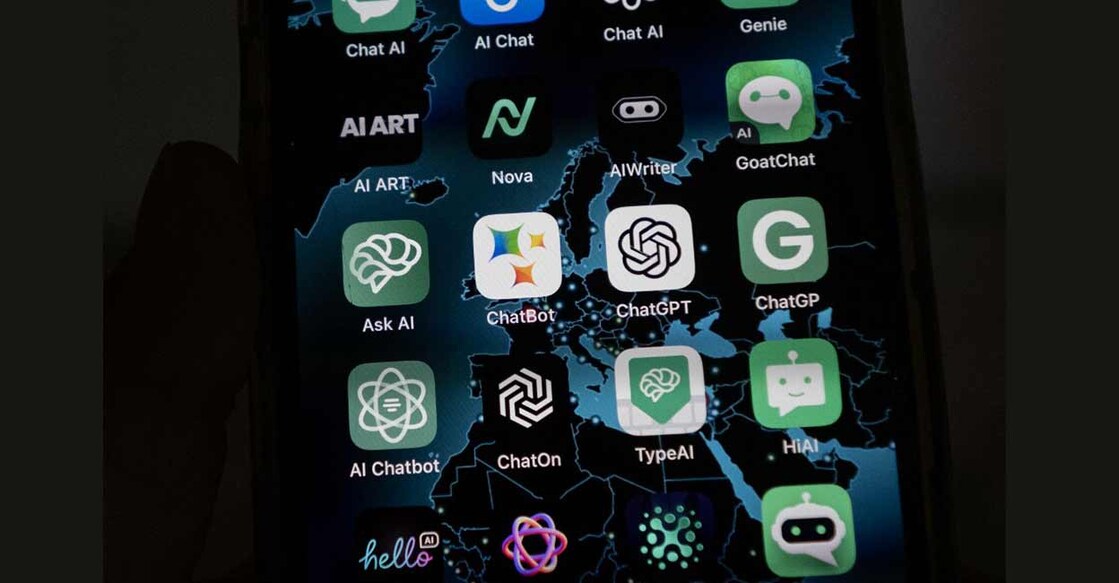
Mail This Article
The United Nations Educational, Scientific and Cultural Organisation (UNESCO) called on governments to regulate the use of Generative Artificial Intelligence (GenAI) in education and research.
Publicly available GenAI tools, such as ChatGPT, can produce automated text, images, videos, music and software code. The platforms have evolved rapidly and are already in use by hundreds of millions around the world, including many students.
Such wide-ranging capacities for information processing and knowledge production have potentially huge implications for education, as they replicate the higher-order thinking that constitutes the foundation of human learning.
However, very few countries have policies in place to ensure safe and ethical use of AI tools.
UNESCO has published global Guidance on Generative AI in Education and Research, designed to address the disruptions caused by GenAI technologies.
UNESCO’s guidance suggests immediate steps that can be taken to ensure a human-centric vision for new technology use.
This includes mandating the protection of data privacy and considering an age limit of 13 for their use in the classroom. It also outlines requirements for GenAI providers for ethical and effective use.
The guidance stresses the need for educational institutions to validate GenAI systems on their ethical and pedagogical appropriateness for education.
From the perspective of a human-centred approach, AI tools should be designed to extend or augment human intellectual abilities and social skills – and not undermine them, conflict with them or usurp them.
What is generative AI?
• Generative AI (GenAI) is an Artificial Intelligence (AI) technology that automatically generates content in response to prompts written in natural language conversational interfaces.
• Rather than simply curating existing webpages, by drawing on existing content, GenAI actually produces new content.
• The content can appear in formats that comprise all symbolic representations of human thinking: texts written in natural language, images (including photographs to digital paintings and cartoons), videos, music and software code.
• GenAI is trained using data collected from webpages, social media conversations and other online media. It generates its content by statistically analysing the distributions of words, pixels
or other elements in the data that it has ingested and identifying and repeating common patterns (for example, which words typically follow which other words).
• While GenAI can produce new content, it cannot generate new ideas or solutions to real-world challenges, as it does not understand real-world objects or social relations that underpin language.
How generative AI works?
The specific technologies behind GenAI are part of the family of AI technologies called Machine Learning which uses algorithms to enable it to continuously and automatically improve its performance from data.
The type of Machine Learning which has led to many of the advances in AI that we have seen in recent years, such as the use of AI for facial recognition, is known as Artificial Neural Networks (ANNs), which are inspired by how the human brain works and its synaptic connections between neurons. There are many types of ANNs.
Text generative AI uses a type of ANN known as a General-purpose Transformer, and a type of General-purpose Transformer called a Large Language Model. This is why AI Text GenAI systems are often referred to as Large Language Models (LLMs). The type of LLM used by text GenAI is known as a Generative Pre-trained Transformer, or GPT (hence the ‘GPT’ in ‘ChatGPT’).
Image GenAI and music GenAI typically use a different type of ANN known as Generative Adversarial Networks (GANs) which can also be combined with Variational Autoencoders. GANs have two parts (two ‘adversaries’) — the ‘generator’ and the ‘discriminator’. In the case of image GANs, the generator creates a random image in response to a prompt, and the discriminator tries to distinguish between this generated image and real images. The generator then uses the result of the discriminator to adjust its parameters, in order to create another image. The process is repeated, possibly thousands of times, with the generator making more and more realistic images that the discriminator is less and less able to distinguish from real images. For example, a successful GAN trained on a dataset of thousands of landscape photographs might generate new but unreal images of landscapes that are almost indistinguishable from real photographs.
Meanwhile, a GAN trained on a dataset of popular music (or even music by a single artist) might generate new pieces of music that follow the structure and complexity of the original music.
GenAI has a myriad of possible uses
The release of ChatGPT in November 2022, followed by iteratively more sophisticated versions, sent shock waves worldwide, and is fuelling the race among large technology companies to position themselves in the field of GenAI model development.
GenAI has a myriad of possible uses. It can automate information processing and the presentation of outputs across all key symbolic representations of human thinking. It enables the delivery of final outputs by furnishing semi-finished knowledge products.
By freeing humans from some categories of lower-order thinking skills, this new generation of AI tools might have profound implications for how we understand human intelligence and learning.
Millions of people are now using GenAI in their daily lives and the potential of adapting the models to domain-specific AI applications seems unlimited, at least in the years to come.
Controversies around generative AI
GenAI raises multiple immediate concerns related to issues such as safety, data privacy, copyright and manipulation. Some of these are broader risks related to artificial intelligence that have been further exacerbated by GenAI, while others have newly emerged with this latest generation of tools.
i) Use of content without consent
GenAI models are built from large amounts of data (for example text, sounds, code and images) often scraped from the Internet and usually without any owner’s permission. Many image GenAI systems and some code GenAI systems have consequently been accused of violating Intellectual Property Rights. There are several ongoing international legal cases that relate to this issue.
ii) AI-generated content polluting the Internet
Because GPT training data is typically drawn from the Internet, which all too frequently includes discriminatory and other unacceptable language, developers have had to implement what they call ‘guardrails’ to prevent GPT output from being offensive and/or unethical. However, due to the absence of strict regulations and effective monitoring mechanisms, biased materials generated by GenAI are increasingly spreading throughout the Internet, polluting one of the main sources of content or knowledge for most learners across the world.
This is especially important because the material generated by GenAI can appear to be quite accurate and convincing, when often it contains errors and biased ideas. This poses a high risk for young learners who do not have solid prior knowledge of the topic in question. It also poses a recursive risk for future GPT models that will be trained on text scraped from the Internet that GPT models have themselves created which also include their biases and errors.
iii) Lack of understanding of the real world
Text GPTs are sometimes pejoratively referred to as ‘Stochastic Parrots’. While they can produce text that appears convincing, that text often contains errors and can include harmful statements. This all occurs because GPTs only repeat language patterns found in their training data (usually text drawn from the Internet), starting with random (or ‘stochastic’) patterns, and without understanding their meaning – just as a parrot can mimic sounds without actually comprehending what it is saying.
The disconnect between GenAI models ‘appearing’ to understand the text that they use and generate, and the ‘reality’ that they do not understand the language and the real world can lead teachers and students to place a level of trust in the output that it does not warrant. This poses serious risks for future education.
iv) Generating deepfakes
GenAI can be used to alter or manipulate existing images or videos to generate fake ones that are difficult to distinguish from real ones. GenAI is making it increasingly easy to create these ‘deepfakes’ and ‘fake news’. GenAI is making it easier for certain actors to commit unethical, immoral and criminal acts, such as spreading disinformation, promoting hate speech and incorporating the faces of people, without their knowledge or consent, into entirely fake and sometimes compromising films.
v) Ethical concerns
GenAI systems in education may exacerbate existing disparities in access to technology and educational resources, further deepening inequities.
GenAI systems in education may reduce human-to-human interaction and the critical social-emotional aspects of learning.
It may limit learners’ autonomy and agency by providing predetermined solutions or narrowing the range of possible learning experiences. Their long-term impact on young learners’ intellectual development needs to be investigated.
GenAI systems that mimic human interactions may have unknown psychological effects on learners, raising concerns about their cognitive development and emotional well-being, and about the potential for manipulation.
As more sophisticated GenAI systems are being developed and applied in education, they are likely to generate new biases and forms of discrimination based on the training data and methods used by the models, which can result in unknown and potentially harmful outputs.